Session 1
Data and data preprocessing
The purpose of this session will be to reintroduce us to the concept of data. We will look at different data types, the important accepts of data that inform the health analytics process, and how we can present our data to our target audience.
Evidence of Learning
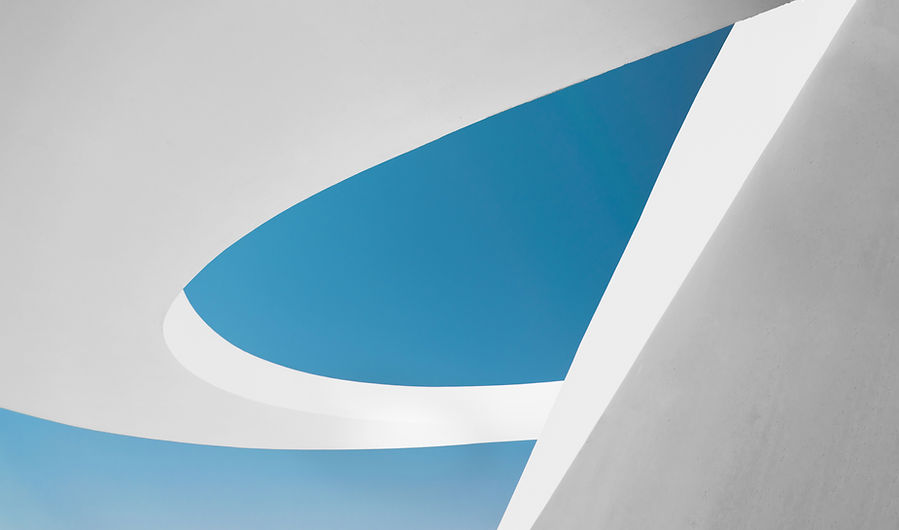
Applying Pandas to a Dataset
01.
Installing and Loading Pandas
pip install pandas
import pandas as pd
df = pd.read_csv('dataset.csv') # Replace 'dataset.csv' with your file path
Evaluating Data Quality
Data quality is a cornerstone of effective analysis and decision-making. If you're working with Python, tools like YData-Profiling and Great Expectations can make evaluating and maintaining data quality a seamless process.
​
YData-Profiling:
What is YData-Profiling?
​
YData-Profiling (formerly Pandas-Profiling) is an excellent tool for automatic exploratory data analysis (EDA), profiling, and even comparing datasets. It can generate detailed insights into your dataset’s structure, distributions, and interactions.
​
Installation and Setup
Install the package using pip:
​pip install ydata_profiling
Then, import the necessary functions in your Python environment:
python:
​
from ydata_profiling import ProfileReport, compare import pandas as pd
​
Creating a Profile Report
To generate a profile report, load your data into a DataFrame and call the profiling functions:
​
data = pd.read_csv("heart.csv") report = ProfileReport(data, title="Heart Disease Data Profile") report.to_file("profile_report.html")
​
Alternatively, view the report directly in a Jupyter Notebook:
​
report.to_notebook_iframe()
​
The resulting report includes:
​
-
Overview: Key dataset statistics, variable distributions, and missing values.
-
Alerts: Warnings on potential data quality issues, such as duplicates or constant columns.
-
Reproducibility Information: Details to replicate the analysis.
​
Handling Large Datasets
For extensive datasets, set the minimal argument to streamline profiling:
​
report = ProfileReport(data, title="Minimal Profile", minimal=True)
You can also specify certain variables for detailed interactions to avoid overloading computations:
\
report.config.interactions.targets = ["RestingBP", "Cholesterol"] report.df = data report.to_file("focused_profile.html")
​
Comparing Datasets
Compare multiple datasets or versions using the compare function:
​
train_report = ProfileReport(train_df, title="Training Data") test_report = ProfileReport(test_df, title="Testing Data") comparison = train_report.compare(test_report) comparison.to_file("comparison.html")
​
YData-Profiling is particularly useful when working with sensitive data, as it operates locally without sending data to external services.
​
Great Expectations: Automating Data Validation
​
What is Great Expectations?
Great Expectations is a robust framework for data validation, offering tools to define and test assumptions about your data. It’s ideal for setting up automated quality checks in data pipelines.
​
Installation and Setup
Install the package using pip:
​
pip install great_expectations
Import the library and load your dataset:
​
import great_expectations as gx data = gx.read_csv("heart.csv")
​
You can also convert a Pandas DataFrame into a Great Expectations object:
​
import pandas as pd data = pd.read_csv("heart.csv") data = gx.from_pandas(data)
Defining Expectations
Expectations are declarative statements about your data. For example:
-
Ensuring columns match a specific set:
​
data.expect_table_columns_to_match_set( column_set=['Age', 'Sex', 'Cholesterol'], exact_match=True )
-
Checking for unique values in a column:
​
data.expect_column_values_to_be_unique(column="id")["success"]
​
-
Verifying data completeness with thresholds:
​
data.expect_column_values_to_not_be_null("Age", mostly=0.80)["success"]
​
Visualising Results
Great Expectations provides a web-based interface, Data Docs, for reviewing validation outcomes. It highlights passed and failed expectations with detailed explanations.