Understanding Reinforcement Learning
- Vusi Kubheka
- Nov 21, 2024
- 4 min read
Reinforcement Learning (RL) represents a powerful branch of machine learning focused on decision-making in uncertain environments (Synopsys.com, n.d.). Rooted in the principles of trial-and-error learning, RL enables systems to identify optimal strategies through interaction with their surroundings, akin to how children learn about the world by experimenting and observing the consequences of their actions (Synopsys.com, n.d.).
What Is Reinforcement Learning?
At its core, RL revolves around an agent learning to achieve a goal by maximising cumulative rewards in an environment. Unlike supervised learning, where labelled data guides the learning process, RL operates independently, discovering the best course of action through experimentation (AWS, n.d.). This involves balancing immediate rewards with the potential for greater delayed rewards - a challenge similar to making decisions in real-life situations where long-term outcomes matter.

How Does Reinforcement Learning Work?
Reinforcement Learning operates under a formal framework borrowed from Markov Decision Processes (MDP) (Synopsys.com, n.d.). The key elements of this system include:
The Agent: The decision-maker exploring the environment.
The Environment: The external system the agent interacts with.
The Policy: A strategy the agent uses to determine its actions.
The Reward Signal: Feedback received for each action, indicating success or failure.
A critical abstraction in RL is the value function, which assesses the 'goodness' of a state not just based on immediate rewards but also on future rewards achievable from that state. The objective of RL is to discover the policy that maximises this value function, guiding the agent toward optimal behaviour.
Types of RL Algorithms
RL algorithms can be broadly classified into model-free and model-based approaches.
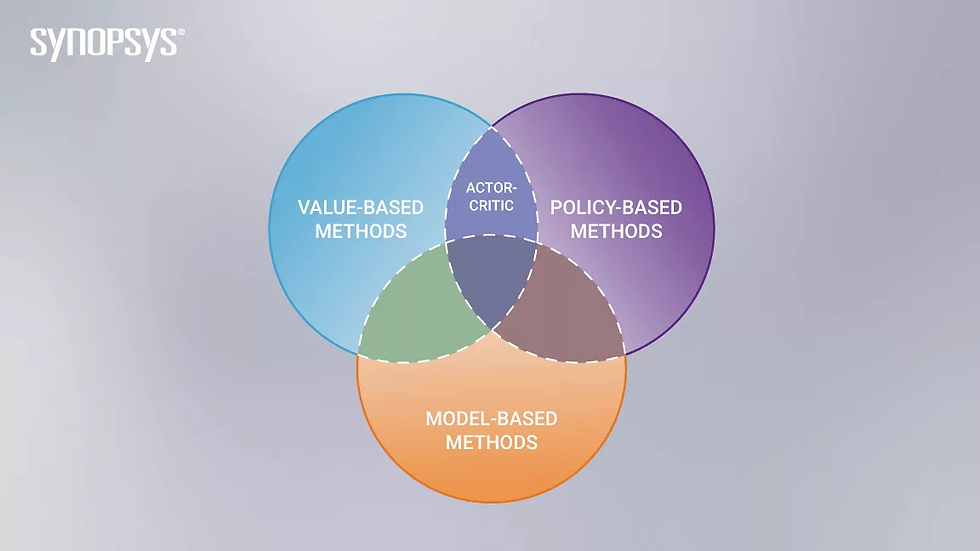
1. Model-Free Algorithms
These do not create an explicit model of the environment. Instead, they learn directly from experience by sampling actions and observing outcomes. Model-free approaches fall into two categories:
Value-Based Algorithms: These estimate the value function for every state using the Bellman equation and derive the optimal policy by acting greedily with respect to these values. Examples include Q-learning and SARSA.
Policy-Based Algorithms: Instead of estimating the value function, these directly optimise the policy by parameterising it with learnable weights. Algorithms such as REINFORCE and deterministic policy gradient (DPG) fall into this category. While effective for continuous action spaces, policy-based methods can suffer from high variance, causing instability during training.
2. Actor-Critic Algorithms
Combining the strengths of both value-based and policy-based approaches, the actor-critic algorithm parametrises both the policy (actor) and the value function (critic). This hybrid approach offers stable training and efficient use of data, making it a popular choice in RL applications.
3. Model-Based Algorithms
These build an explicit model of the environment by predicting outcomes (rewards and states) for every action. This allows the agent to plan its strategy without interacting directly with the environment, simulating decision-making processes akin to human thought experiments.
Applications of Reinforcement Learning
The adaptability of RL has unlocked its potential across diverse industries. Below are some noteworthy applications:
1. Robotics
Robots operating in unpredictable environments—such as navigating unknown terrain or handling novel objects—benefit immensely from RL. It enables them to adapt dynamically, making RL a key technology for robotic path planning and motion control (Scribbr, 2023).
2. Autonomous Driving
Self-driving vehicles rely on RL to handle complex tasks like path planning, motion prediction, and decision-making in real-time. RL’s ability to learn from uncertain environments is critical for ensuring safe and efficient autonomous navigation (Synopsys.com, n.d.).
3. AlphaGo and Gaming
RL has revolutionised strategic gameplay. AlphaGo, an RL-powered agent, mastered the ancient game of Go by playing against itself and professional players. Its success demonstrates RL’s capacity to outperform human expertise in complex decision-making scenarios (Synopsys.com, n.d.).
4. Healthcare
In personalised medicine, RL is applied to design dynamic treatment regimes (DTRs). By analysing patient data, RL systems develop tailored treatment plans for chronic illnesses, optimising long-term health outcomes (Scribbr, 2023).
5. Education
Adaptive learning systems powered by RL personalise tutoring experiences, identifying student weaknesses and tailoring content delivery to improve educational outcomes (Scribbr, 2023).
6. Natural Language Processing (NLP)
Text summarisation, machine translation, and predictive text applications integrate RL to optimise language processing tasks by learning from feedback loops (Scribbr, 2023).
Benefits of Reinforcement Learning
RL stands out for its versatility and ability to solve complex problems. Its key benefits include:
Excelling in Complex Environments: RL thrives in scenarios with dynamic rules and dependencies where human-designed solutions fall short (Synopsys.com, n.d.).
Reducing Human Intervention: Unlike traditional ML models requiring labelled datasets, RL learns independently, while still accommodating human feedback when necessary (Synopsys.com, n.d.).
Optimising for Long-Term Goals: RL’s focus on cumulative rewards makes it ideal for applications requiring strategic foresight and delayed gratification (Synopsys.com, n.d.).
Challenges and Future of RL
Despite its promise, RL faces challenges such as high computational demands, sample inefficiency, and instability in training. However, advancements in computational power and algorithmic design continue to push the boundaries of RL applications (Synopsys.com, n.d.). As RL evolves, its potential to solve complex, real-world problems will only grow, transforming industries and redefining the capabilities of artificial intelligence.
References
Scribbr. (2023, July 14). What are some real-life applications of reinforcement learning? https://www.scribbr.com/frequently-asked-questions/what-are-some-real-life-applications-of-reinforcement-learning/
What is Reinforcement Learning? - Reinforcement Learning Explained - AWS. (n.d.). Amazon Web Services, Inc. https://aws.amazon.com/what-is/reinforcement-learning/
What is Reinforcement Learning? – Overview of How it Works | Synopsys. (n.d.). https://www.synopsys.com/glossary/what-is-reinforcement-learning.html
コメント